Anyone involved in last year’s exam grade saga probably harbours a level of resentment against algorithms.
The government formula was designed to standardise grades across the country. Instead, it affected students disproportionately, raising grades for students in smaller classes and more affluent areas. Conversely, students in poorer performing schools had their grades reduced, based on past grades from previous years.
Most of us are well versed in the chaos that followed. Luckily, the government have already confirmed that this year’s results will be mercifully algorithm-free.
We touched on the increased use of AI in education in an article last year. Simple algorithms are already used to mark work in online learning platforms. Other systems can trawl through the websites people visit and the things that they write, looking for clues about poor mental health or radicalisation. Even these simple systems can create problems, but the future brings machine learning algorithms designed to support detailed decision making with major impacts on peoples lives. Many see Machine Learning as an incredible opportunity for efficiency, but it is not without its controversies.
Image-generation algorithms have been the latest to cause issues. A new study from Carnegie Mellon University and George Washington University, found that unsupervised machine learning led to ‘baked-in biases’. Namely, the assumption that women simply prefer not to wear clothes. When researchers fed the algorithm pictures of a man cropped below his neck, 43% of the time the image was auto completed with the man wearing a suit. Researchers also fed the algorithm similarly cropped photographs of women. 53% of the time, it auto completed with a woman in a bikini or a low-cut top.
In a more worrying example of machine-learning bias, A man in Michigan was arrested and held for 30 hours after a false positive facial recognition match. Facial recognition software has been found to be mostly accurate for white males but, for other demographics, it is woefully inadequate.
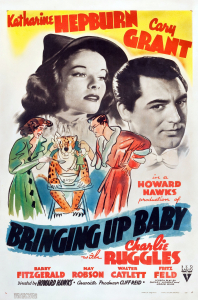
Starring Cary Grant and Katherine Hepburn, Bringing up Baby follows a palaeontologist through his adventures with a scatter-brained heiress… and a leopard called Baby.
Where it all goes wrong:
These issues arise because of one simple problem, garbage in, garbage out. Machine learning engines take mountains of previously collected data, and trawl through them to identify patterns and trends. They then use those patterns to predict or categorise new data. However, feed an AI biased data, and they’ll spit out a biased response.
An easy way to understand this is to imagine you take German lessons twice a week and French lessons every other month. Should someone talk to you in German, there’s a good chance you’ll understand, and be able to form a sensible reply. However, should someone ask you a question in French, you’re a lot less likely to understand, and your answer is more likely to be wrong. Facial recognition algorithms are often taught with a white leaning dataset. The lack of diversity means that when the algorithm comes across data from another demographic, it can’t make an accurate prediction.
Coming back to image generation, the reality of the internet is that images of men are a lot more likely to be ‘safe for work’ than those of women. Feed that to an AI, and it’s easy to see how it would assume women just don’t like clothes.
AI in Applications:
While there’s no denying that being wrongfully arrested would have quite an impact on your life, it’s not something you see every day. However, most people will experience the job application process. Algorithms are shaking things up here too.
Back in 2018, Reuters reported that Amazon’s machine learning specialists scrapped their recruiting engine project. Designed to rank hundreds of applications and spit out the top five or so applicants, the engine was trained to detect patterns in résumés from the previous ten years.
In an industry dominated by men, most résumés came from male applicants. Amazon’s algorithm therefore copied the pattern, learning to lower ratings of CVs including the word “women’s”. Should someone mention they captain a women’s debating team, or play on a women’s football team, their resume would automatically be downgraded. Amazon ultimately ended the project, but individuals within the company have stated that Amazon recruiters did look at the generated recommendations when hiring new staff.
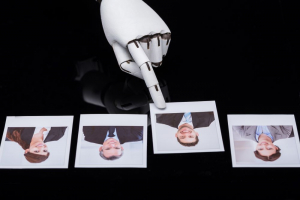
Algorithms are already in use for recruitment. Some sift through CVs looking for keywords. Others analyse facial expressions and mannerisms during interviews.
Protection from Automated Processing:
Amazon’s experimental engine clearly illustrated how automated decision making can drastically affect the rights and freedoms of individuals. It’s why the GDPR includes specific safeguards against automated decision-making.
Article 22 states that (apart from a few exceptions), an individual has the right not to be subject to a decision based solely on automated processing. Individuals have the right to obtain human intervention, should they contest the decision made, and in most cases an individual’s explicit consent should be gathered before using any automated decision making.
This is becoming increasingly important to remember as technology continues to advance. Amazon’s experiment may have fallen through, but there are still AI-powered hiring products on the market. Companies such as Modern Hire and Hirevue provide interview analysis software, automatically generating ratings based on an applicant’s facial expressions and mannerisms. Depending on the datasets these products were trained on, these machines may also be brimming with biases.
As Data Controllers, we must keep assessing the data protection impact of every product and every process. Talking to wired.co.uk, Ivana Bartoletti (Technical Director–Privacy at consultancy firm Deloitte) stated that she believed the current Covid-19 pandemic will push employers to implement AI based recruitment processes at “rocket speed”, and that these automated decisions can “lock people out of jobs”.
Battling Bias:
We live in a world where conscious and unconscious bias affects the lives and chances of many individuals. If we teach AI systems based on the world we have now, it’s little wonder that the results end up the same. With the mystique of a computer generated answer, people are less likely to question it.
As sci-fi fantasy meets workplace reality (and it’s going to reach recruitment in schools and colleges first) it is our job to build in safeguards and protections. Building in a Human based check, informing data subjects, and completing Data Protection Impact Assessments are all tools to protect rights and freedoms in the battle against biased AI.
Heavy stuff. It seems only right to finish with a machine learning joke:
A machine learning algorithm walks into a bar…
The bartender asks, “What will you have?”
The algorithm immediately responds, “What’s everyone else having?”
The technologies used to process person data are becoming more sophisticated all the time.
This is the first article of an occasional series where we will examine the impact of emerging technology on Data Protection. Next time, we’ll be looking at new technologies in the area of remote learning.